The digital landscape has forever transformed the way we shop and do business. If you’re old enough to remember dial up internet, scratchy connections and MySpace pages, today’s eCommerce ecosystem might seem like something straight out of science fiction. While we can’t log on to buy a flying car (yet!) today’s eCommerce technology is nothing short of space age.
We are living in interesting times, with online shoppers outstripping offline, but that’s not to say success is a given. According to the 2023 State of eCommerce report[1], “The pandemic accelerated changes that were already underway in eCommerce. Consumers shop differently now. They are less brand loyal. They expect more and if they’re not initially satisfied, they will go elsewhere to find the products and experiences they want.”
Fortunately, there is a way to navigate this space and emerge successfully on the other side.
Behavioural analytics, deep learning, and real-time analytics provide online retailers with a level of knowledge and insight that could only have been dreamt of as little as three or four years ago. But these are the very technologies that can equip eCommerce businesses with the data, insight and understanding needed to encourage customer loyalty, offer enhanced experiences, and reduce churn.
Behavioural Analytics
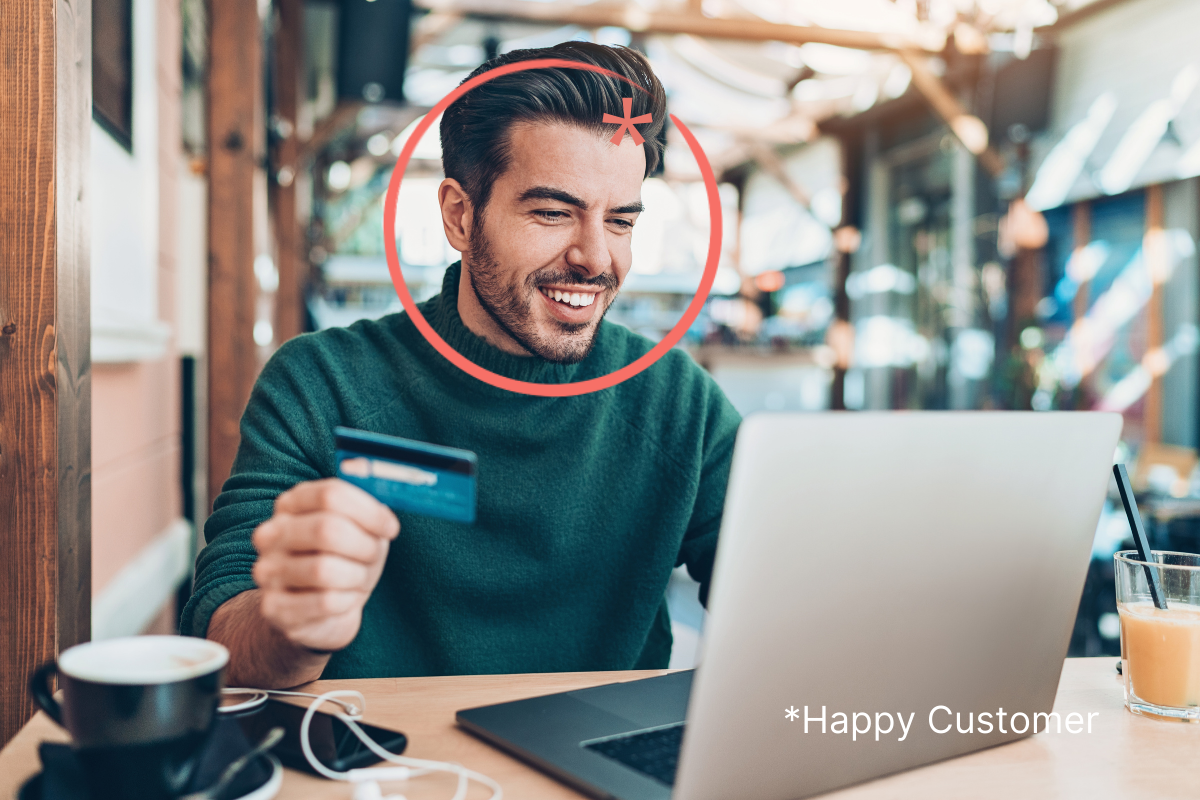
Envision a world where businesses can effortlessly decode the intricate tapestry of customer behaviour. Well, you don’t have to imagine. It exists. Enter behavioural analytics—a game-changer for eCommerce enterprises seeking to unlock the true potential of their customer interactions.
Behavioural analytics is a powerful tool that enables businesses to delve deep into the intricacies of customer behaviour. By meticulously tracking and analysing customer interactions, businesses gain valuable insights into how customers engage with their services.
Using behavioural analytics, businesses can tailor their marketing strategies and offerings to cater to the specific needs and preferences of distinct groups of customers based on the data derived from their behaviour. This targeted approach ensures that marketing efforts are more effective, as they are precisely aimed at the audience most likely to respond positively.
Decathlon, for instance, used QUIN Audience Engine, to gain real-time insights into the intentions of its visitors.
By leveraging predictive capabilities and taking proactive measures, the retailer successfully reduced the rate of journey abandonment from 24% to 16% without compromising the average basket size and profit margins. This improvement was achieved by strategically implementing a threshold for free delivery, which was only offered for orders above a specific basket size.
Moreover, behavioural analytics facilitates the prediction of user behaviour, especially in dynamic environments such as online marketplaces. In the rapidly evolving eCommerce landscape, where user behaviour can change in an instant, understanding and accurately predicting customer actions become critical.
Companies can also use behavioural analytics to gain insights into individual preferences and make accurate recommendations based on those insights. This level of personalisation enhances the customer experience, as customers are presented with products that align closely with their preferences.
Amazon, the eCommerce giant, has transformed the online shopping experience by harnessing the true power of behavioural analytics. As you navigate through its virtual aisles, you are greeted by a personalised homepage carefully tailored to your unique preferences, demographics, past purchases, search queries, and even the products you have previously viewed.
Behind the scenes, a treasure trove of data – gleaned from behavioural analytics - drives this exceptional level of personalisation, enabling Amazon to tailor its platform to suit your needs and desires. This wealth of data allows Amazon to create a customised shopping experience that feels as though it was crafted just for you.
Real-Time Analytics
In this era of instant gratification and real-time connectivity, businesses cannot afford to lag behind. Real-time analytics emerges as the knight in shining armour, allowing businesses to analyse data as it is generated—a real-time symphony of insights. This capability for providing live insights is essential for improving eCommerce procedures and staying ahead in a competitive market.
One key technique within real-time analytics is online learning, where Machine Learning models are continuously updated as new data streams in. This approach ensures that the models stay current and adapt to changing user behaviour promptly.
In the context of eCommerce, where user behaviour can shift rapidly, online learning plays a vital role in accurately predicting and understanding customer actions. By constantly updating ML models with fresh data, businesses can capture the latest trends, and thus make agile decisions and take proactive measures.
Another technique that complements real-time analytics is sequential pattern analysis. This technique is a structured approach to data mining, which focuses on patterns of events that happen concurrently.
By analysing data as it flows in, businesses can identify any patterns or anomalies that might impact their eCommerce approach. Sequential mining enables businesses to respond promptly to emerging patterns or shifts in consumer behaviour, ensuring that they can adapt their offerings in real-time.
With the combination of online learning and sequential mining, businesses can gain a comprehensive understanding of user behaviour and leverage that knowledge for various purposes. For instance, real-time analytics can help optimise inventory management by predicting demand patterns and ensuring the availability of popular products.
Deep Learning and Advanced Analytics Techniques
Deep learning, powered by neural networks such as convolutional neural networks (CNNs) and recurrent neural networks (RNNs), has transformed the field of predictive analytics, particularly in real-time applications. These advanced algorithms excel in analysing sequential data, making them highly effective in understanding user clickstreams and predicting behaviour in eCommerce settings.
Originally designed for image processing and computer vision tasks, CNNs have proven to be versatile in examining sequences of data as well. In the context of user behaviour analysis, CNNs can analyse sequences of user interactions with a website or mobile app, such as clicks, swipes, and taps. By leveraging the hierarchical structure of CNNs, businesses can extract meaningful features from the sequential data and gain an understanding of user behaviour in real-time.
For example, an eCommerce website can utilise a CNN to evaluate a sequence of user interactions with its product images and use the results to personalise the product recommendations shown to the user in real-time. This personalised approach enhances the user experience, which increases the likelihood of conversion.
In contrast, Recurrent Neural Networks (RNNs) are specialised for analysing sequential data that evolves over time, such as click patterns from users or written text. They allow companies to anticipate the next step a user might take by considering their past actions and the circumstances surrounding those actions. For example, a social media site could use an RNN to anticipate which post a user is likely to interact with next, taking into account their previous interactions on the platform and the currently popular content.
Ensemble learning, which combines multiple Dachine Learning models, is another tool that contributes to the real-time prediction of user behaviour, and is particularly effective in dynamic environments where data is constantly changing.
By leveraging diverse models with complementary strengths, ensemble learning enhances prediction accuracy and robustness. This tool can enable businesses to capture the complex and evolving dynamics of user behaviour, resulting in more accurate predictions and better decision-making.
Clustering techniques also play a significant role in the prediction of user behaviour. This involves grouping similar data points together, allowing businesses to categorise distinct user segments based on how they interact with their platforms.
By training Deep Learning models to group users based on their behaviour patterns, companies can gain insights into how different groups of users are likely to behave in the future. This information can be invaluable for tailoring their approach to marketing and designing personalised experiences.
Conclusion
To thrive in the eCommerce industry, businesses must embrace advanced analytics and make data-driven decisions that cater to their customers' evolving needs and preferences. If you’re looking to leverage the power of advanced analytics, Quin can help you bring each of these technologies together to create a useful, detailed and accurate understanding of your users.. With advanced customer analytics at your fingertips, you can make data-driven decisions, adapt quickly to changing user behaviour, and stay ahead of your competitors. Want to see how deep learning can increase your conversion rate? Get in touch with one of our experts today.
To Read the whole post